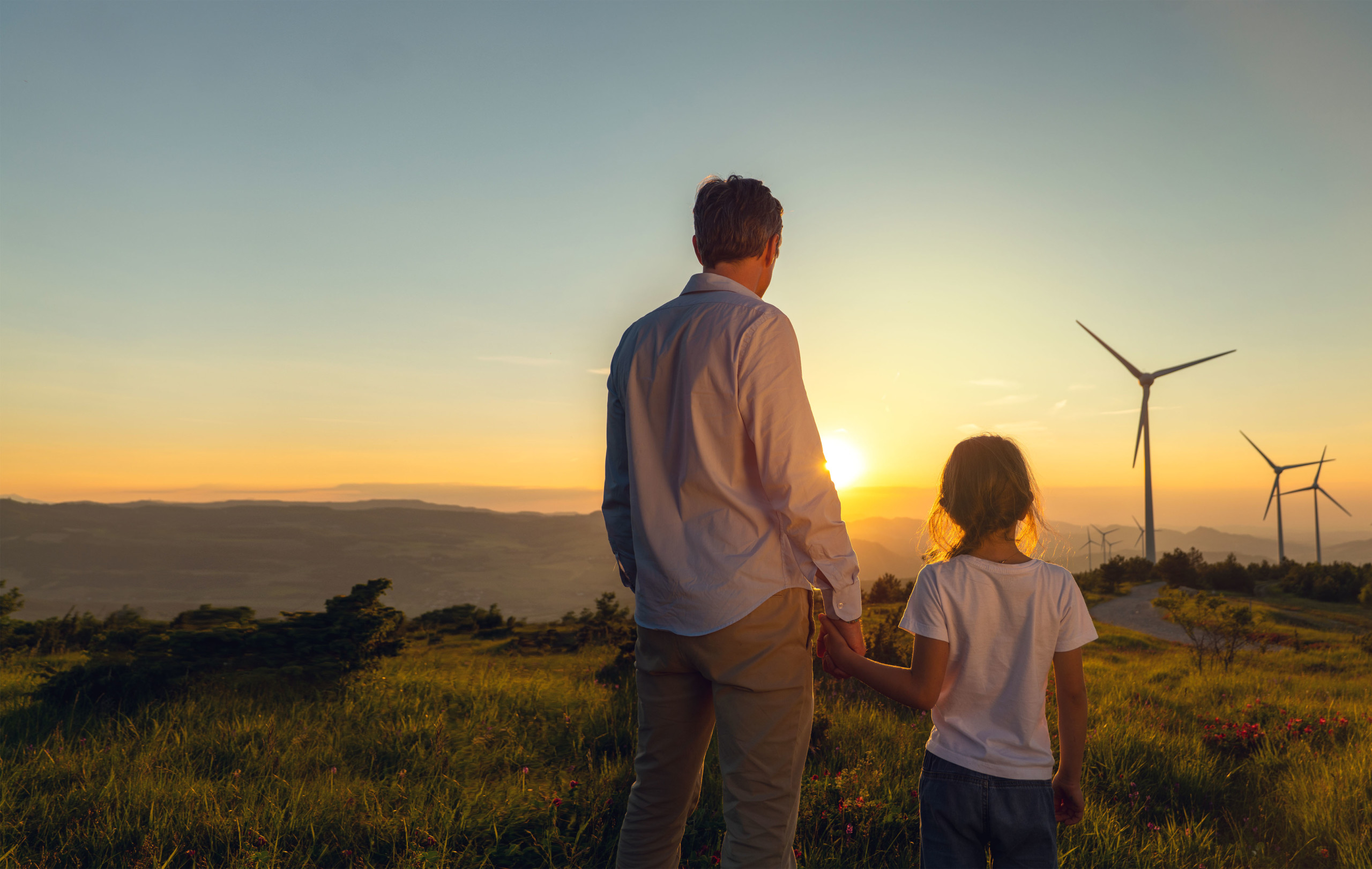
The future delivered. Seamlessly.
Meaningful impact for societies and businesses, through smart transformation and elimination of costly friction.
We create seamless experiences that are human, intelligent, valuable, and resilient. We help people, governments, and companies navigate an increasingly complex world.
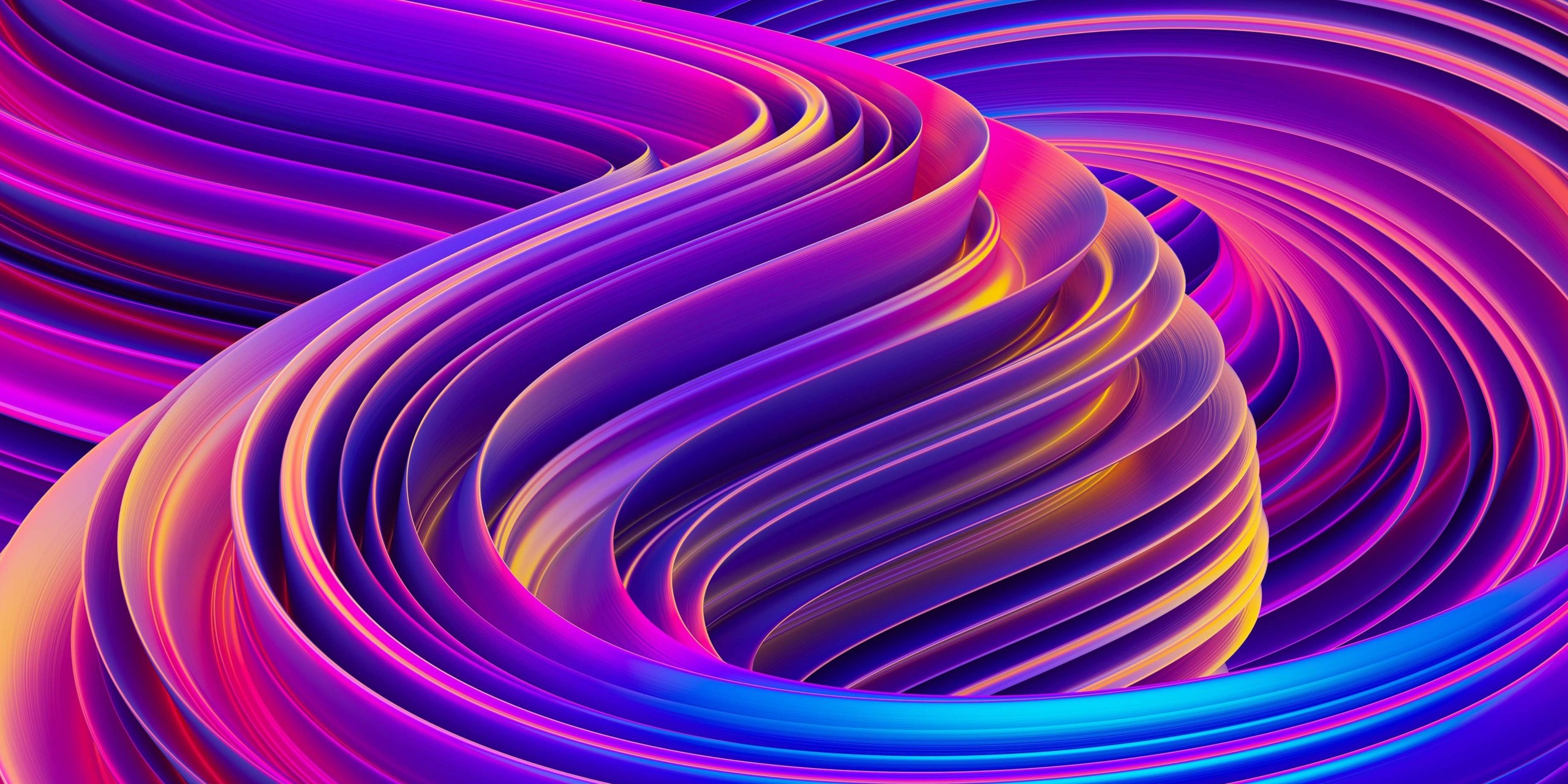
Creating Seamless Experiences
Traditional approaches to transformation are failing in uncertain and volatile environments. Meanwhile, adaptability, speed to market, and diverse thinking are becoming ever more critical to success. At Nortal, we utilize our proven seamless experience methodology to deliver world-class solutions without the pitfalls of traditional transformation methods.
Industries
Together with our customers, we are redefining how businesses, governments, and healthcare institutions operate.
Public Sector
The right digital tools applied properly can dramatically transform citizen-government interaction, changing the way societies work. We provide end-to-end transformations, starting from scratch and providing service over the entire product life. We speak policy and strategy. We consult, draft legislation, provide impact analysis, design organizations, build capacity, develop cyber resilience, and create digital products and services, all of which culminate in value for citizens.
Learn moreHealthcare
An aging population coupled with higher expectations from patients means governments and healthcare providers struggle to offer access to affordable and high-quality healthcare. Our digital healthcare solutions fundamentally transform doctor-patient interactions, while making more efficient use of taxpayers’ resources.
Learn moreFinancial Services and Insurance
Change in the financial services sector is constantly accelerating at every level: with customer behavior, macroeconomics, as well as regulatory and technological levels. We help finance organizations build and modernize their core technology and services for scalability, agility, and regulatory compliance, while meeting the changing security, data governance, and quality policies.
Learn moreIndustry
Digitized business means employing data to increase turnover, improve production, reduce waste, and allow expansion to new business models. We are forerunners in leveraging operational technology with hybrid cloud and data-driven solutions in manufacturing, logistics, and construction.
IndustryEnergy and Resources
Technology is ushering energy industries into a new era. Automation leads to lower costs, new efficiencies, sustainability, and productivity. Our pace-layered approach brings value beyond solving the immediate problem, creating backbone solutions to handle massive numbers of transactions, creating differentiation, and giving our clients a competitive edge, all the while supporting innovation and new services.
Learn moreConsumer
The modern consumer is not easy to satisfy. Whatever your electronic platform, seamless interactions are now a requirement for both internal and external users. Our wide variety of industry experience in digital commerce, coupled with extreme depth in interoperability, electronic identity, and service design, puts us in a unique position to offer flexible and agile solutions that scale with your business.
Learn moreTelecommunications, Media and Entertainment
For over 20 years we’ve worked with the world’s top telecommunication companies to create and implement new products into both back-end technology and customer-facing storefronts. Our certified engineers and professionals are not believers in one-size-fits-all products, and our full-lifecycle, holistic approach has delivered custom solutions to help our clients thrive in one of the world’s most competitive sectors.
Learn moreTwo decades of leading transformations
20+
years of experience – We’ve partnered with leading businesses and governments to attack their most mission-critical challenges for over 20 years.
40%
We delivered 40% of Estonia’s e-transformation and executed countless other digital government projects around the world.
100+
transformation projects – Our track record is more than 100 transformation projects for industry leaders. In North America alone, we serve 25+ Fortune 500 customers in telecommunications, media, and more.
Selected work
We are proud to work with some of the most influential organizations in the marketplace.
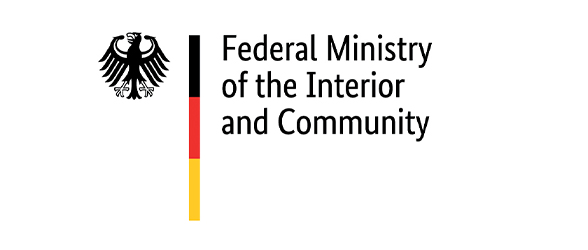
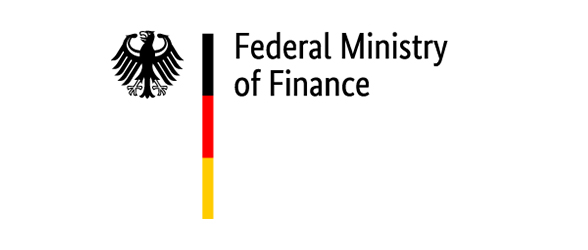
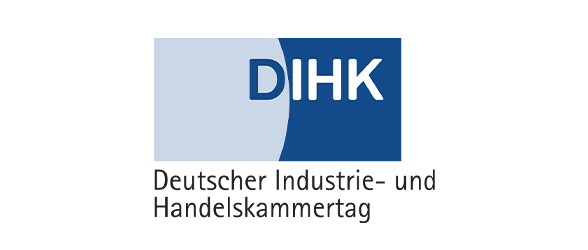
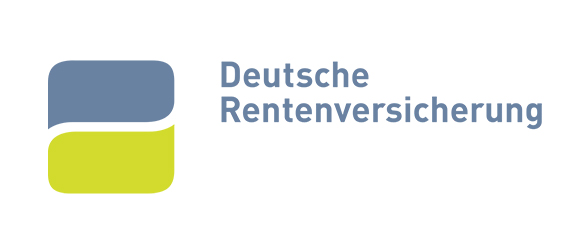
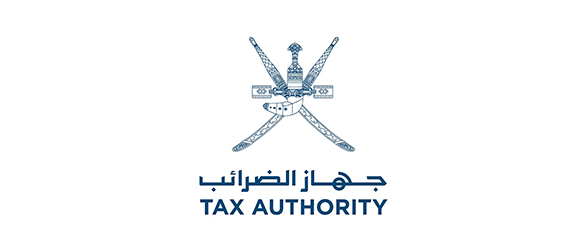
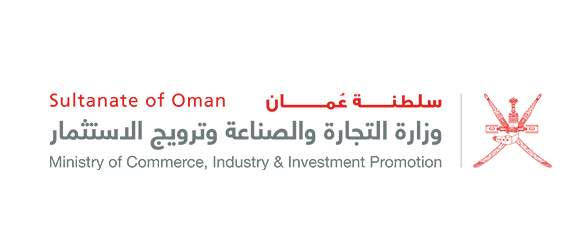
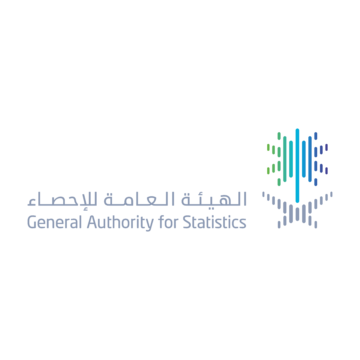
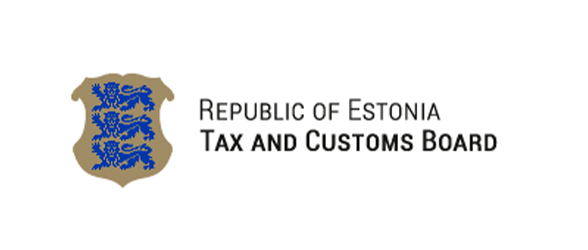
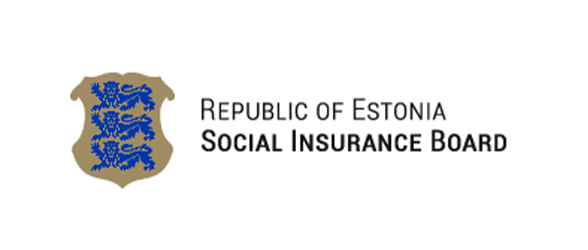


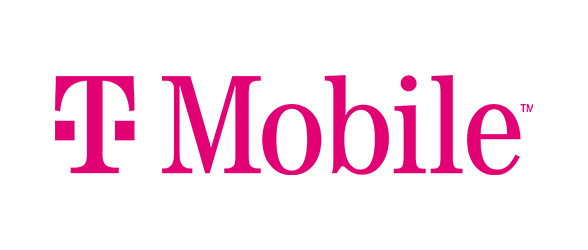
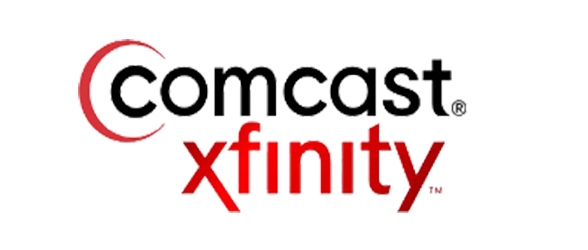
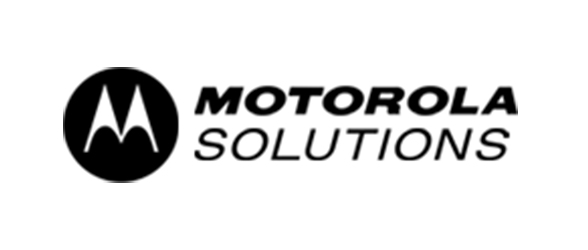
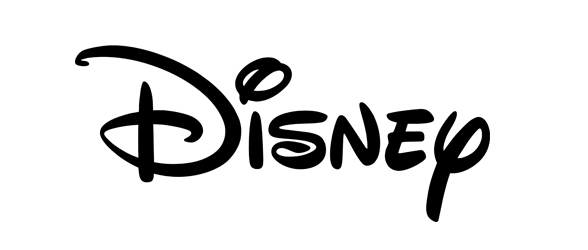
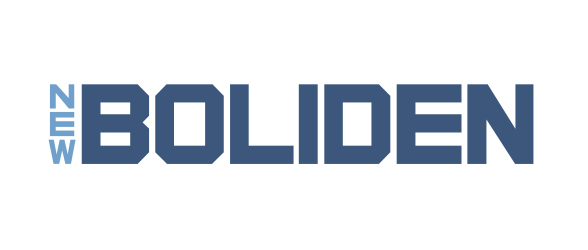

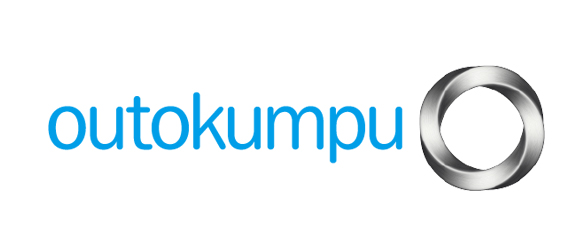
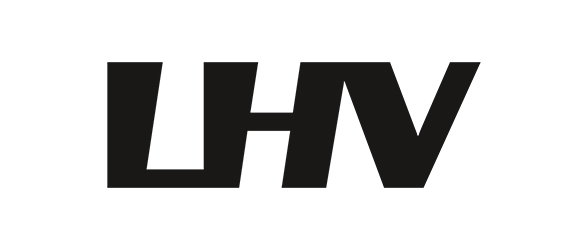
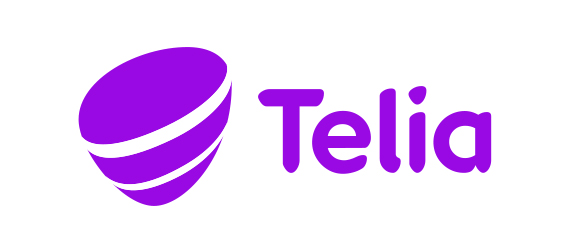
Get in touch
Would you like to discuss a project? We’d love to hear from you.